Zip Code – All zip codes within Delaware, OH however roads with no zip codes were manually populated by their location.
Recorded Document – datasets contain points that are recorded documents in Delaware County. It was made to help find missing documents in the County.
School District – This set contains all school districts in Delaware County and was created for the Auditor’s parcel records.
Map Sheet – Contains the map sheet of Delaware County.
Farm Lot – All farm lots in the Virginia Military and US military for the Survey Districts.
Township – To identify the geographic boundaries of each township.
Street Centerline – The center of private and public roads. Used for 911 emergency response, appraisal mapping, accident reports, disaster management.
Annexation – All Delaware County annexations and conforming boundaries from 1853.
Condo – Dataset consists of condominiums polygons in Delaware County.
Subdivision – All subdivisions and condoms recorded in Delaware County Recorder’s office.
Survey – The survey points are a shapefile of a point coverage that represents all surveys of land. All surveys were scanned and saved as a pdf file.
Dedicated ROW – all dedicated road right of way polygons in Delaware County.
Tax Districts – Data set has all tax districts in Delaware County, Ohio.
GPS – identifies all GPS monuments set between 1991 and 1997 the Coordinates are in Universal Transverse Mercator Northing and Easting.
Original Township – all original boundaries of the townships before the tax district changed .
Address Points (DXF) – The State of Ohio Location Based Response Systems (LBRS) address points show a spatially accurate placement of address in a parcel.
Precinct – Polygons that determine each voting boundary.
Hydrology – all major waterways in Delaware County.
Building Outline 2021 – all building outlines of structures.
Parcel – all parcels in Delaware County.
PLSS – all polygons depicting the boundaries of 2 public military land survey districts.
Street Centerlines (DXF) – The State of Ohio LBRS street centerlines are the center [pavement of all private and public roads.
Address Point – The State of Ohio LBRS address points data are spatially accurate representation of all certified addresses.
2022 Leaf-On Imagery (SID file) – includes 2021 Imagery (SID file), Township, Original Township, and Recorded Document.
Delaware County Contours – 2018 2ft contours for the county is a geodatabase format.
Delaware County E911 Data – The layer can reverse geocode a set of coordinates to determine the closest valid address for 911 agencies.
Address Points – layer is intended to support appraisal mapping, and 911 Emergency Response.
Street Centerlines – layer is intended to support appraisal mapping, and 911 Emergency Response.
2021 Imagery (SID File) – contains 2022 Leaf-On-Imagery (SID file), zip code, recorded document, dedicated ROW.
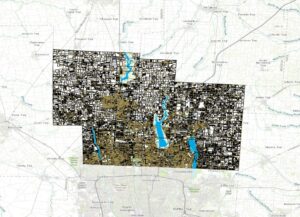